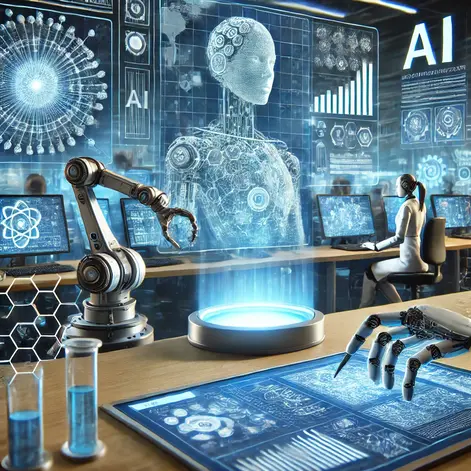
Sakana AI’s AI Scientist system is an advanced AI research model designed to autonomously handle the entire scientific research process—from ideation to execution and paper writing. The successful peer review of an AI-generated paper signals a potential transformation in how academic research is conducted, paving the way for a new era of AI-assisted discoveries in various fields.
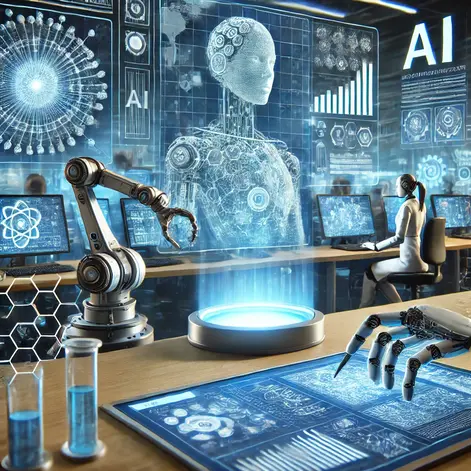
AI Scientist: Revolutionizing Research with Artificial Intelligence
The AI Scientist developed by Sakana AI is an advanced system capable of autonomously generating novel research ideas, writing code, conducting experiments, summarizing findings, and composing research papers. Unlike traditional AI tools that assist human researchers, this system operates with minimal human intervention, making it one of the first AI models to function as an independent scientific researcher.
A key component of AI Scientist is its automated peer-review mechanism, which utilizes another large language model (LLM) to assess the originality and quality of its own research papers. This self-regulating system ensures that the AI-generated work meets rigorous academic standards, making it suitable for publication in reputed scientific conferences and journals.
Sakana AI’s Paper Acceptance at ICLR 2025
For its debut in academic publishing, Sakana AI submitted three research papers created by AI Scientist to the ICLR 2025 workshop. One of these papers successfully passed the stringent peer-review process and was formally accepted. The research focused on advancements in AI model architecture, optimization techniques, and machine learning efficiency.
The peer-review process for ICLR is known to be highly competitive, with reviewers critically evaluating submissions for originality, validity, and contribution to the field. The acceptance of an AI-generated paper demonstrates that AI can not only conduct high-level research but also meet the strict evaluation criteria set by the scientific community.
Implications for the Future of AI in Research
The success of AI Scientist in generating a peer-reviewed paper raises important questions about the role of AI in academic research. Some experts believe that AI could accelerate scientific breakthroughs by automating time-consuming research processes. This would allow human scientists to focus on higher-level conceptual thinking and ethical considerations while AI handles data-intensive tasks.
However, concerns remain regarding authenticity, accountability, and ethical implications of AI-generated research. The potential for AI to fabricate data, manipulate results, or introduce biases needs to be addressed before AI-driven research can become mainstream. Additionally, academic institutions and publishing bodies will need to develop new guidelines and ethical frameworks to regulate AI-generated academic work.
The acceptance of the world’s first AI-generated research paper by a major academic conference is a landmark moment in AI-driven scientific innovation. Japanese startup Sakana AI has not only showcased the capabilities of autonomous AI research models but also initiated a broader conversation about how AI will shape the future of academia, research, and knowledge generation.
As AI continues to evolve, the boundaries between human-led and AI-generated research will continue to blur, prompting global discussions on ethics, credibility, and the role of artificial intelligence in science. While this breakthrough is an exciting glimpse into the future, the world must now carefully navigate the challenges that come with AI-powered academic advancements.
Sources-