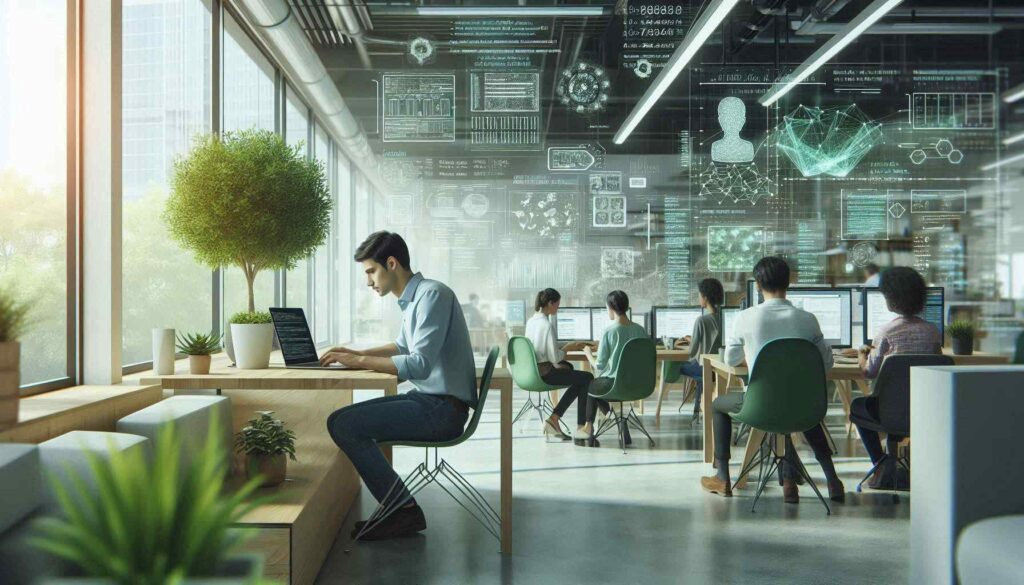
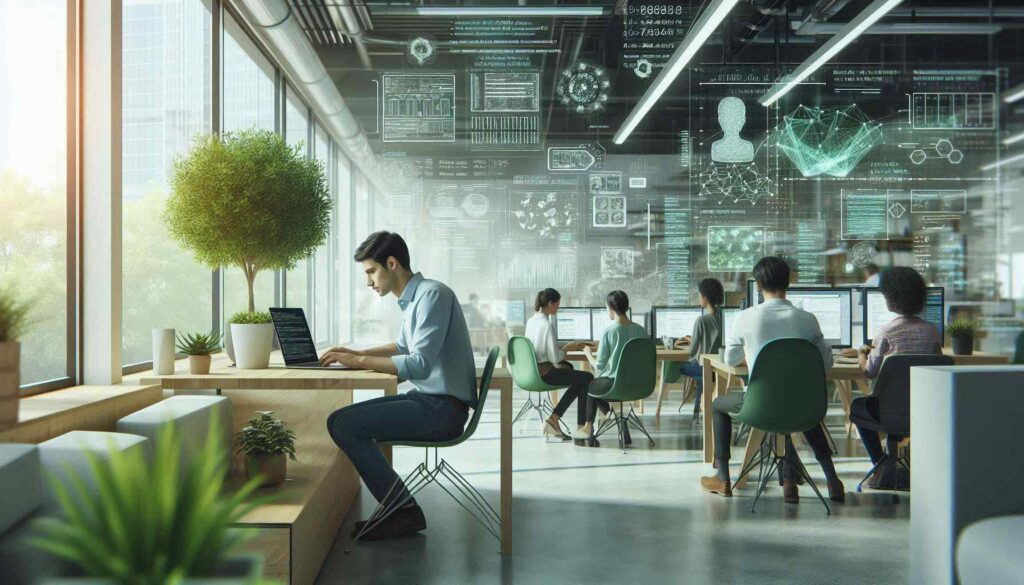
The Importance of AI Model Research Certification
1. Enhancing Skills and Knowledge
AI model research certification equips professionals with critical skills and knowledge essential for developing, evaluating, and optimizing AI models. Here are some key areas that certification programs cover:
Statistical Analysis
A strong foundation in statistics is vital for effective AI model research. Participants learn about:
- Data Distributions: Understanding how data is distributed helps in selecting appropriate models and evaluating their performance.
- Hypothesis Testing: Formulating and testing hypotheses ensures that AI models are grounded in sound evidence.
- Regression Analysis: This technique is fundamental for predicting outcomes and understanding the relationships between variables, allowing researchers to make informed decisions.
Machine Learning Algorithms
A deep dive into machine learning (ML) algorithms is a core component of AI model research certification. Candidates explore:
- Supervised Learning: This area focuses on developing models that predict outcomes based on labeled input data, covering algorithms like linear regression and decision trees.
- Unsupervised Learning: Understanding clustering and dimensionality reduction techniques help uncover patterns in unlabeled data, enabling researchers to gain insights that might otherwise remain hidden.
- Reinforcement Learning: This exciting area trains models to make decisions through trial and error, essential for applications in robotics and game playing.
Model Evaluation Techniques
Effective model evaluation is crucial for ensuring reliability. Candidates learn:
- Performance Metrics: Understanding precision, recall, F1 score, and ROC-AUC allows professionals to assess model accuracy comprehensively.
- Cross-Validation: Techniques like k-fold cross-validation ensure that models generalize well to unseen data, reducing the risk of overfitting.
- A/B Testing: This method enables practitioners to compare model performances in real-world scenarios, optimizing AI applications continuously.
2. Meeting Industry Demand
The demand for AI professionals is skyrocketing, with a projected growth rate of 22% from 2020 to 2030, according to the U.S. Bureau of Labor Statistics. Various sectors, including healthcare, finance, and technology, are eager to employ individuals with expertise in AI model research. A report by McKinsey highlights that 70% of organizations are expected to adopt at least one AI application by 2030, creating an urgent need for skilled professionals. By obtaining certification, individuals position themselves favorably within this expanding job market, demonstrating their commitment to mastering the latest technologies and methodologies.
3. Building Credibility and Trust
Certification in AI model research signals to potential employers that the individual possesses a specific level of knowledge and competence in the field. This credential enhances resumes and builds trust among peers and employers, serving as a testament to the individual’s dedication to ongoing learning and professional development. Many hiring managers view certifications as indicators of a candidate’s seriousness about their career and readiness to tackle complex AI challenges.
Key Competencies Developed Through Certification
AI model research certification enhances knowledge and equips candidates with competencies essential for success. Here are some key competencies:
1. Data Management Skills
Managing large datasets is crucial in AI research. Certification programs typically cover:
- Data Cleaning: Techniques for preprocessing and cleaning data ensure it is suitable for analysis.
- Data Wrangling: Skills to manipulate and transform raw data into a format usable for modeling are emphasized.
- Database Management: Understanding how to use databases and data warehouses for efficient data storage and retrieval is fundamental for data-driven decision-making.
2. Programming Proficiency
Most AI model research certifications emphasize programming skills, particularly in languages and tools such as:
- Python: The go-to programming language for AI and ML due to its simplicity and extensive libraries like TensorFlow and PyTorch.
- R: Known for its statistical computing capabilities, R is often used in data analysis and visualization.
- SQL: Essential for querying databases, SQL is crucial for managing data and ensuring data quality.
3. Critical Thinking and Problem Solving
AI model research requires strong analytical skills. Certification programs encourage:
- Problem Identification: Learning to identify the right problems to solve with AI solutions.
- Creative Solutions: Encouraging innovative thinking to design novel algorithms and models that can address real-world challenges.
- Adaptability: Training professionals to adjust their approaches based on new findings or changing project requirements.
4. Collaboration and Communication Skills
AI model research often involves teamwork. Certification programs foster:
- Interdisciplinary Collaboration: Understanding how to work with professionals from diverse fields such as data science, software engineering, and business.
- Effective Communication: Learning to articulate complex AI concepts to non-technical stakeholders, ensuring everyone is aligned on project goals.
Emerging Trends in AI Model Research Certification
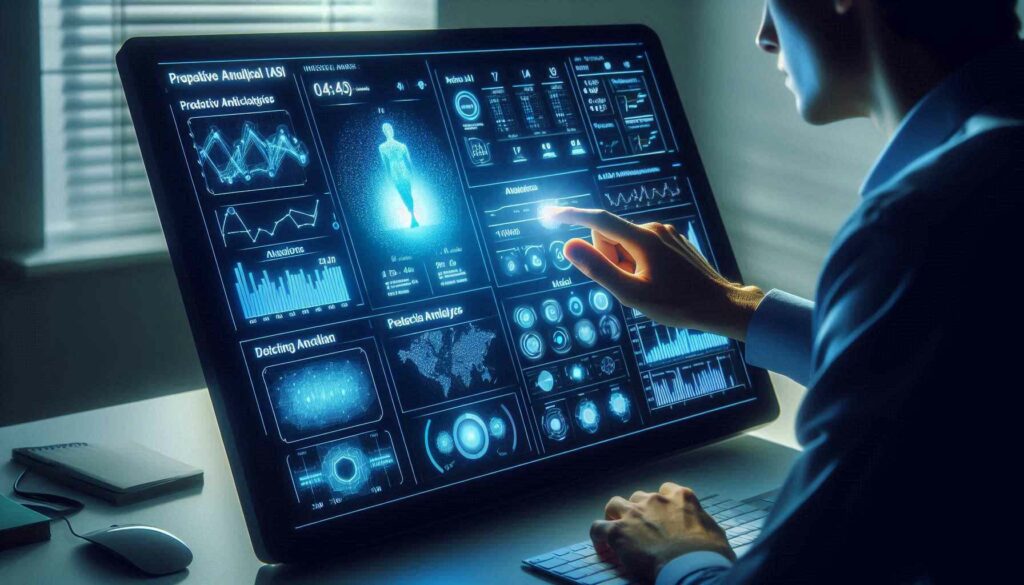
As AI evolves, so do the competencies required for success. Here are some exciting trends shaping the future of AI model research certification:
1. Explainable AI (XAI)
As AI systems are increasingly integrated into decision-making processes, the demand for transparency is growing. Explainable AI (XAI) focuses on making AI models interpretable to users, ensuring they can understand how decisions are made. Certification programs incorporating XAI principles prepare professionals to create models that perform well while providing clear insights into their functioning. Understanding XAI is especially crucial in regulated industries, such as healthcare and finance, where accountability is paramount.
2. Federated Learning
Federated learning is revolutionizing how AI models are trained. This technique allows models to be trained across multiple decentralized devices while keeping data localized. By enhancing data privacy and security, federated learning is particularly relevant in sectors where sensitive data is prevalent. As organizations prioritize data protection, certification programs covering federated learning techniques will become increasingly valuable, enabling professionals to design models that respect user privacy.
3. Transfer Learning
Transfer learning focuses on applying knowledge gained from one domain to another, allowing for efficient model training with limited data. This approach is especially beneficial in scenarios where data collection is challenging or expensive. By understanding transfer learning, professionals can significantly enhance the performance of AI applications across various industries, and certification programs are beginning to emphasize this skill.
4. AI Ethics and Governance
As AI technologies proliferate, ethical considerations and governance frameworks are becoming integral to AI model research. Certification programs increasingly incorporate modules on ethical AI practices, teaching professionals how to identify and address potential biases in their models. Understanding ethical implications is vital for building trust in AI systems, as organizations face heightened scrutiny regarding their AI practices.
The Role of Community in AI Model Research
Engagement with the AI community is crucial for ongoing learning and professional growth. Here’s how involvement can benefit those pursuing certification:
1. Collaborative Learning
Platforms like GitHub and Kaggle provide opportunities for collaboration on AI projects. Participating in competitions and sharing code fosters a collaborative environment where individuals can learn from one another and gain practical experience. Engaging in community-driven projects allows learners to apply theoretical knowledge to real-world challenges, enhancing their skill set.
2. Networking Opportunities
Attending AI conferences, webinars, and meetups fosters connections with industry leaders and peers. Networking can lead to mentorship opportunities, job referrals, and collaborations on research projects. Building a robust professional network is essential in the AI field, where relationships often drive career advancements.
3. Open Source Contributions
Contributing to open-source AI projects can enhance a professional’s portfolio and demonstrate their commitment to the field. Many organizations value candidates who actively participate in the community and contribute to developing open-source AI tools and frameworks.
4. Continuous Learning
The field of AI is ever-evolving. Engaging with the community provides access to the latest research, tools, and best practices. Staying connected with thought leaders and industry experts helps professionals stay informed and relevant in their careers.
Exciting Case Studies: AI Model Research in Action
1. Healthcare Advancements
One compelling example of AI model research is its application in healthcare. A team of researchers developed an AI model capable of predicting patient deterioration in hospitals. By analyzing historical patient data, the model identifies patterns that indicate potential complications, allowing healthcare professionals to intervene earlier. This research not only showcases the power of AI in improving patient outcomes but also emphasizes the importance of certified professionals in developing and deploying such life-saving technologies.
2. Financial Fraud Detection
In the financial sector, AI model research has led to significant advancements in fraud detection systems. One notable case involved a major bank implementing a machine-learning model to identify fraudulent transactions in real time. By analyzing transaction patterns and user behavior, the model effectively reduced fraud rates by over 30%. Certified professionals played a crucial role in the development and continuous improvement of this system, highlighting the practical applications of their skills in safeguarding financial assets.
3. Smart City Solutions
AI model research is also driving innovation in urban planning and smart city initiatives. For instance, a city implemented an AI model to optimize traffic flow by analyzing real-time traffic data. The model dynamically adjusts traffic signals to reduce congestion and improve commute times. This project demonstrates how certified AI professionals contribute to sustainable urban development and enhance the quality of life for residents.
Popular AI Model Research Certification Programs
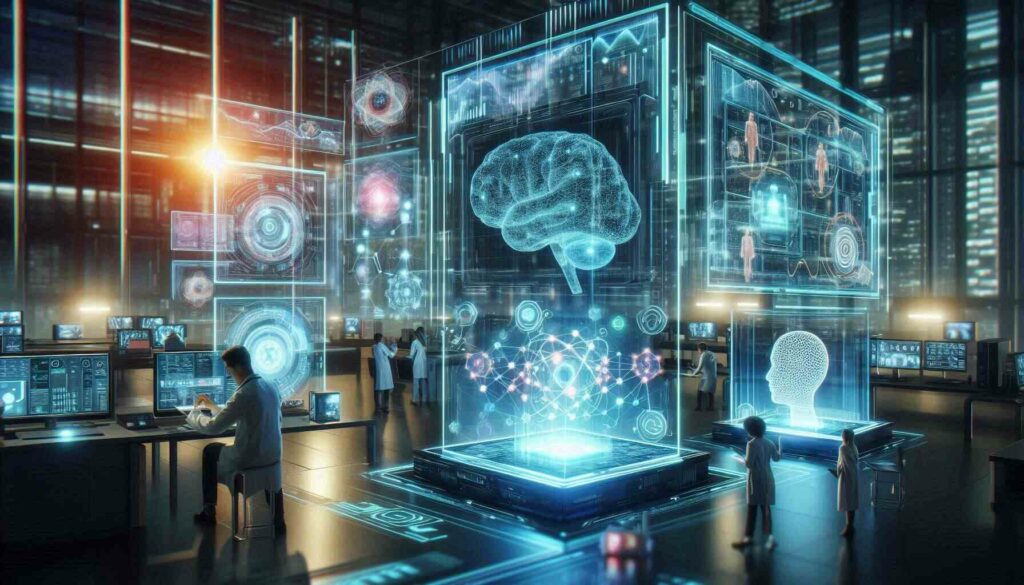
As the AI landscape evolves, various institutions offer certifications tailored to equip professionals with the necessary skills and knowledge. Here are some of the most reputable programs:
1. AI+ Researcher Certification from AI CERTs
The AI+ Researcher Certification from AI CERTs is designed for professionals seeking to advance their knowledge in AI model development and applied research. The program focuses on mastering cutting-edge research methodologies, AI ethics, and building scalable AI solutions. It provides hands-on experience and equips learners with the expertise needed to navigate complex AI challenges in business and academia. Learn more.
2. Google Professional Machine Learning Engineer
This certification validates the ability to design, build, and productionize ML models. The program emphasizes best practices and real-world applications, ensuring that candidates possess a strong foundation in machine learning principles. Learn more.
3. Microsoft Certified: Azure AI Engineer Associate
This certification focuses on developing AI solutions on Microsoft Azure. Candidates learn to integrate AI into applications, manage data, and implement AI solutions, making it ideal for those working within the Microsoft ecosystem. Learn more.
4. IBM AI Engineering Professional Certificate
IBM’s program covers a wide range of topics, including deep learning, reinforcement learning, and natural language processing. Graduates emerge with a robust understanding of AI model development and implementation, equipping them for various AI roles. Learn more.
5. Data Science Council of America (DASCA) AI Model Research Certification
This certification focuses on AI model development and data science best practices. It emphasizes hands-on experience and practical applications, preparing professionals for success in AI model research roles. Learn more.
Conclusion
As AI continues to shape the future of technology, AI model research certification plays a crucial role in equipping professionals with the necessary skills to navigate this dynamic landscape. By understanding the importance of certification, exploring emerging trends, and engaging with the AI community, individuals can position themselves for success in an ever-evolving field. The future of AI model research is bright, and those who invest in their education and certification will undoubtedly play a significant role in shaping the AI revolution.
“Have questions? Ready to explore certifications that drive your career forward? Reach out to AI CERTs today your future in AI excellence awaits!”