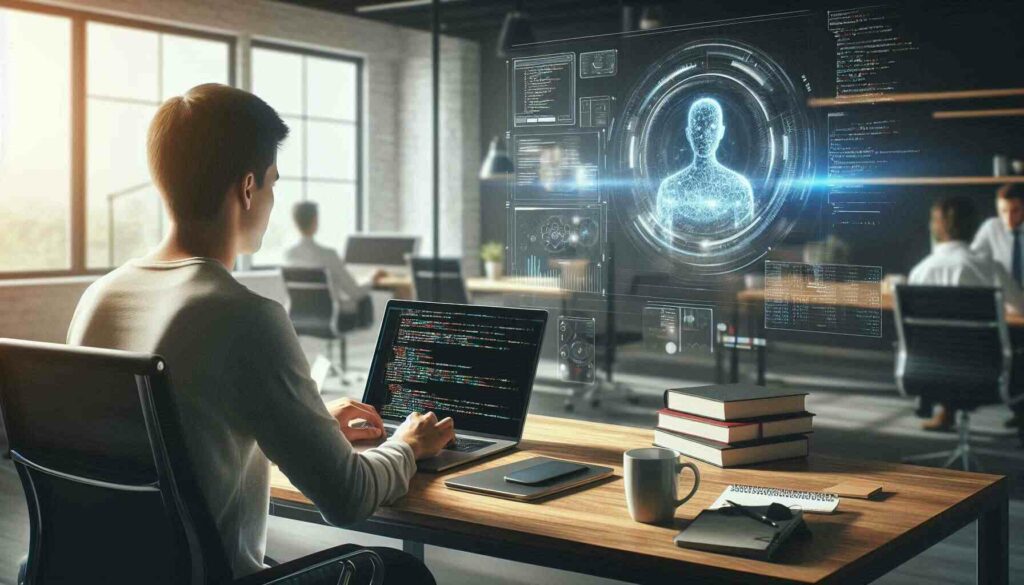
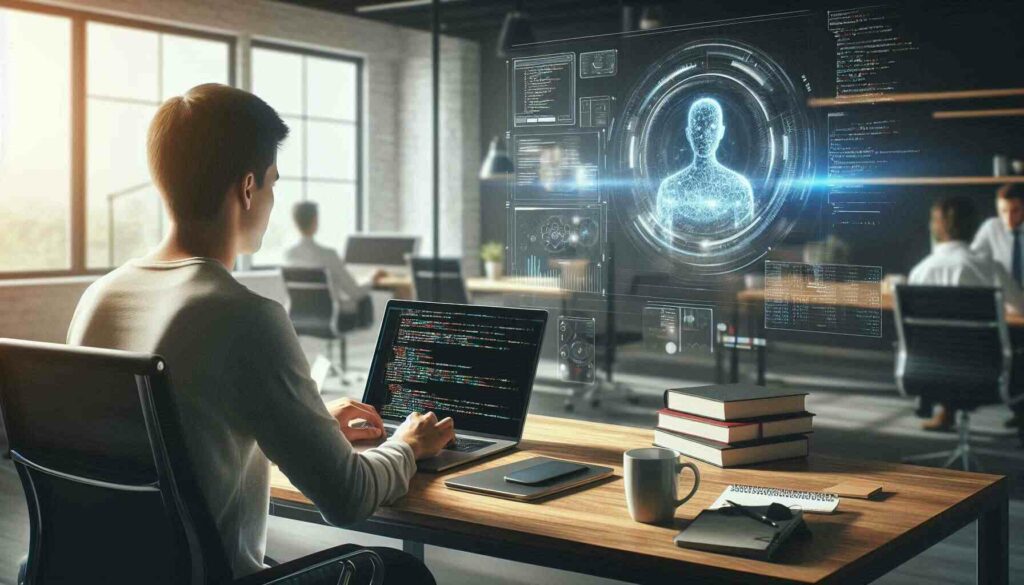
This article dives into what AI engineering is, the skills developers need, the tools to master, real-world applications, and how to get started in this field. If you’re a developer looking to elevate your career, AI Engineering for Developers offers the roadmap you need.
What is AI Engineering for Developers?
At its core, AI Engineering for Developers focuses on integrating artificial intelligence techniques into software systems to create smarter, adaptive solutions. Unlike traditional software engineering, which relies on predefined rules and static code, AI engineering revolves around building systems that learn, predict, and make decisions based on data.
Think of it as the intersection of software development, machine learning, and data science. From chatbots that understand human language to algorithms that recommend personalized content, AI Engineering for Developers enables the creation of systems that improve over time.
Why AI Engineering for Developers Matters Today
The impact of AI is everywhere, from businesses leveraging it to automate tasks to improving customer experiences and gaining insights from data. For developers, this means endless opportunities to create solutions that weren’t possible before.
AI isn’t just a trend, it’s the backbone of the next wave of technological evolution. According to reports, the global AI market is projected to exceed $190 billion by 2025. Developers skilled in AI Engineering for Developers will be at the forefront of this transformation, shaping how industries operate and innovate.
Key Skills for AI Engineering for Developers
To thrive in AI Engineering for Developers, professionals need a mix of programming expertise, mathematical knowledge, and a good understanding of machine learning concepts.
Here are the essential skills:
- Programming Proficiency: Python is the go-to language for AI, thanks to libraries like TensorFlow and PyTorch. R, Java, and C++ are also valuable.
- Mathematics and Statistics: A solid grasp of linear algebra, calculus, and probability is necessary for understanding AI algorithms.
- Machine Learning Knowledge: Developers should know supervised learning, unsupervised learning, and neural networks.
- Data Handling Skills: Expertise in data cleaning, analysis, and tools like SQL or Pandas is crucial.
- Cloud Computing: Familiarity with platforms like AWS, Google Cloud, and Azure is important for deploying AI models.
Mastering these skills is a key step toward becoming proficient in AI Engineering for Developers.
AI Engineering vs. Traditional Software Engineering
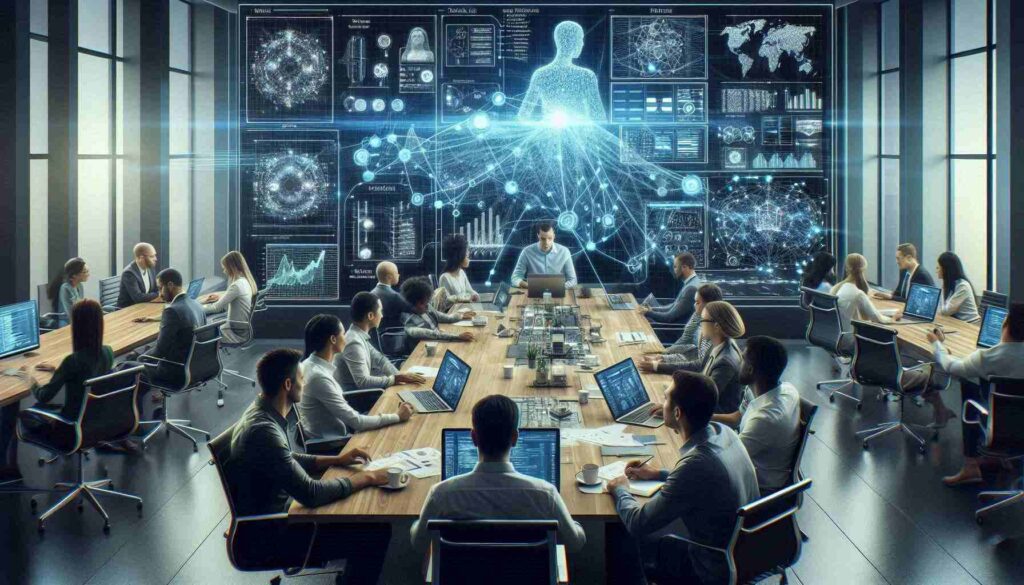
While both roles involve building software, the focus and methods differ significantly.
AI Engineering for Developers | Traditional Software Engineering |
Focuses on creating adaptive systems | Builds static, rule-based systems |
Relies on data-driven algorithms | Relies on predefined logic |
Uses machine learning and AI tools | Uses conventional coding frameworks |
AI Engineering for Developers empowers developers to solve complex problems autonomously, making it a vital skill for the future.
Must-Know Tools for AI Engineering for Developers
A strong grasp of AI tools is crucial for success. Here are some essential technologies for developers:
- TensorFlow: Google’s open-source library for machine learning and AI.
- PyTorch: Known for its flexibility, this tool is widely used in research and production.
- Keras: A high-level API that simplifies neural network development.
- Scikit-learn: Perfect for data analysis and machine learning on smaller datasets.
- Jupyter Notebooks: A tool for writing and testing AI code interactively.
- NVIDIA CUDA: Enhances AI performance through GPU acceleration.
Familiarity with these tools is indispensable for developers working in AI Engineering for Developers.
Real-World Applications of AI Engineering for Developers
The versatility of AI Engineering for Developers has led to innovations across numerous industries:
- Healthcare: AI models assist doctors in diagnosing diseases and predicting patient outcomes.
- Finance: Fraud detection, algorithmic trading, and credit scoring are powered by AI.
- Retail: AI personalizes shopping experiences and optimizes inventory management.
- Customer Service: Virtual assistants and AI chatbots enhance customer interactions.
These applications highlight the vast potential of AI Engineering for Developers to revolutionize industries and improve efficiency.
How to Start in AI Engineering for Developers
Breaking into this field might seem daunting, but it’s achievable with the right steps:
- Learn Programming: Begin with Python, the most popular language in AI.
- Take Online Courses: Platforms like Coursera, Udemy, and edX offer beginner-to-advanced AI programs.
- Build Projects: Develop AI tools, participate in Kaggle competitions, or contribute to open-source AI projects.
- Join AI Communities: Engage with developers on GitHub, Reddit, and LinkedIn for networking and insights.
- Earn Certifications: Boost your credibility with certifications tailored to AI Engineering for Developers.
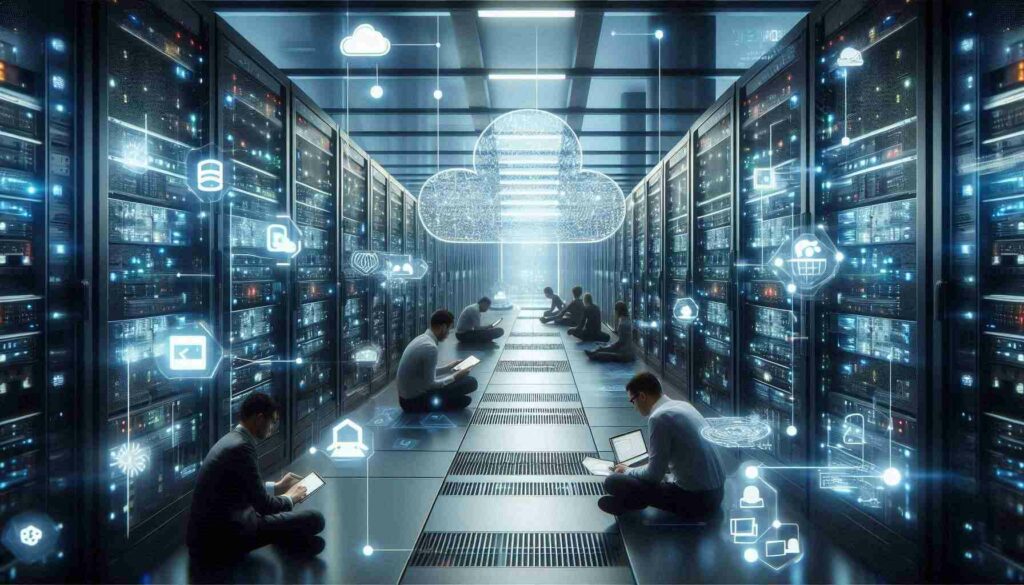
Top Certifications for AI Engineering for Developers
Certifications play a crucial role in validating expertise, especially in cutting-edge fields like AI Engineering for Developers. Whether you’re new to AI or looking to deepen your knowledge, the following certifications can set you apart in a competitive job market.
1. AI Engineering™ by AI CERTs
The AI Engineering™ certification by AI CERTs is a premier program for developers seeking to master AI from foundational concepts to advanced deployment techniques. This certification covers:
- Machine Learning Foundations: Understanding supervised, unsupervised, and reinforcement learning.
- Data Handling and Preprocessing: Techniques for cleaning, analyzing, and preparing datasets.
- Model Deployment: Best practices for deploying AI models in production environments.
- Optimization Techniques: Improving AI model efficiency and scalability.
- AI Ethics and Bias Mitigation: Addressing ethical considerations and fairness in AI systems.
Benefits:
- Industry-recognized credential to enhance career prospects.
- Access to a community of AI professionals for networking and support.
- Hands-on projects to showcase real-world skills.
2. Machine Learning by Stanford University (Coursera)
This is a highly recommended course for developers, taught by Andrew Ng, a leading figure in AI and machine learning. The program offers:
- Foundational Concepts: Linear regression, logistic regression, and neural networks.
- Machine Learning Techniques: Clustering, anomaly detection, and recommender systems.
- Algorithm Implementation: Practical coding assignments in MATLAB or Octave.
- Mathematical Insights: Building a strong mathematical foundation for AI applications.
Benefits:
- Ideal for beginners transitioning to AI engineering.
- Flexible, self-paced online learning.
- Certificate issued by Stanford University, boosting credibility.
3. Microsoft Azure AI Engineer Associate
This certification is tailored for developers leveraging Azure’s AI services to build intelligent applications. Core areas include:
- AI Solution Design: Integrating AI services such as Cognitive Services and Azure Bot Services.
- Machine Learning Model Deployment: Using Azure Machine Learning to deploy, manage, and monitor AI models.
- Data Insights: Leveraging Azure Synapse Analytics for data integration and analysis.
Benefits:
- Certification demonstrates expertise in cloud-based AI engineering.
- Hands-on labs to prepare for real-world challenges.
- Access to Microsoft’s AI ecosystem and exclusive tools.
4. Google Cloud Professional Data Engineer
This certification is designed for developers focusing on data-driven AI solutions. The program emphasizes:
- Data Processing Pipelines: Building scalable and efficient data workflows.
- AI Model Deployment: Operationalizing AI systems on Google Cloud.
- Machine Learning Applications: Using BigQuery ML and Vertex AI for AI model creation.
- Performance Optimization: Techniques to enhance system reliability and speed.
Benefits:
- Demonstrates ability to handle complex data and AI challenges.
- Opens doors to roles in cloud and AI-focused companies.
- Access to Google Cloud resources for continued learning.
5. IBM AI Engineering Professional Certificate
IBM’s program provides a hands-on learning experience for developers eager to dive into AI engineering. It features:
- AI Fundamentals: Introduction to machine learning, deep learning, and neural networks.
- Hands-on Projects: Building applications with TensorFlow, Keras, and Scikit-learn.
- Natural Language Processing: Creating NLP solutions for text and language-based applications.
- AI in Action: Real-world use cases like fraud detection and image recognition.
Benefits:
- A comprehensive curriculum ideal for developers at all levels.
- Gain proficiency in widely used AI tools and frameworks.
- Certificate from IBM, a globally trusted brand.
Investing in these certifications equips developers to excel in AI Engineering for Developers.
The Future of AI Engineering for Developers
The future is bright for AI Engineering for Developers, with advancements expected in quantum computing, augmented reality, and autonomous systems. Developers specializing in AI engineering will play a critical role in integrating these technologies into real-world solutions.
The demand for AI engineers is growing, and developers with expertise in AI Engineering for Developers will remain highly sought after. The opportunities are endless, and now is the time to seize them.
Conclusion
AI engineering is not just a skill, it’s a gateway to innovation. For developers, mastering AI Engineering for Developers means building systems that solve real-world problems, adapt to change, and shape the future of technology.
Ready to get started? Explore the AI+ Engineer™ by AI CERTs and begin your journey in AI today. Contact us now.