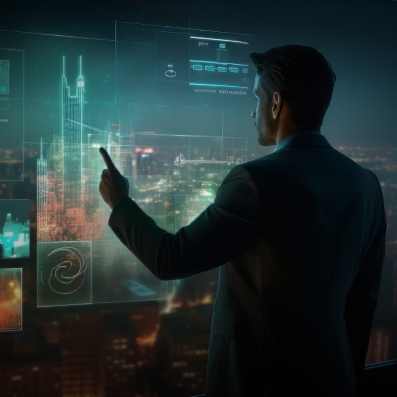
AI Legal Compliance
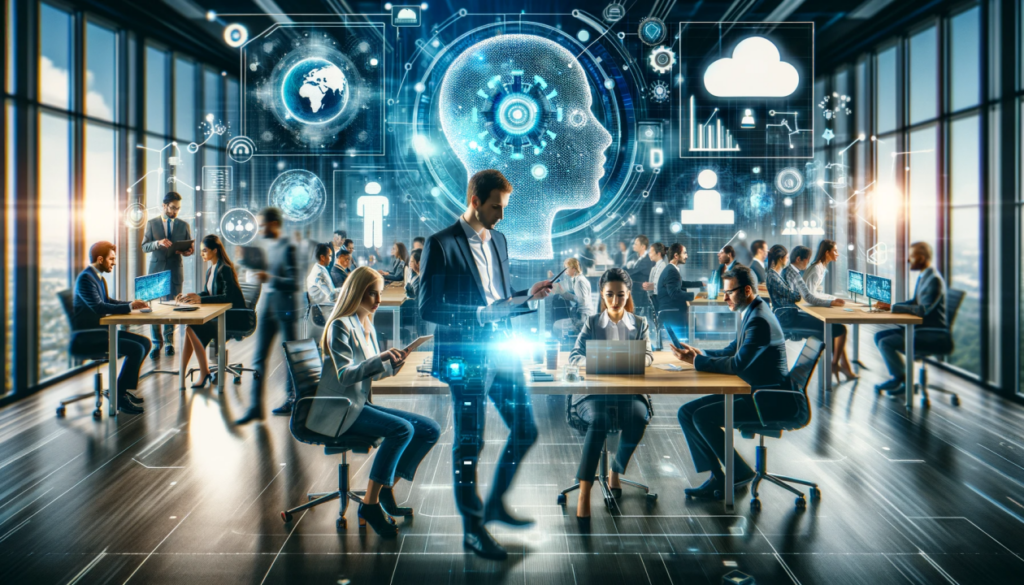
The rapid development of Artificial Intelligence (AI) has brought immense benefits across various industries. However, alongside these advancements lie legal and regulatory challenges that businesses must navigate. A recent study by PWC revealed that a staggering 72% of executives are concerned about these challenges, highlighting the need for robust AI governance solutions. The global AI governance market is expected to reach a staggering $10.7 billion by 2027, reflecting the growing demand for compliance measures.
Here’s a breakdown of some key areas of focus within AI legal compliance:
Data Privacy
AI revolves around data collection, storage, and analysis where issues related to data breaches have been observed. While stringent regulations like GDPR and CCPA exist, stricter enforcement is crucial to ensure robust data privacy protections.
Algorithmic Bias
AI algorithms are only as fair as the data they’re trained on. Unfortunately, bias can creep in at various stages: from skewed datasets to programmer assumptions and even the metrics used to evaluate success. This can lead to discriminatory outcomes, favoring specific groups unintentionally. To combat this, fairness audits and mitigation techniques are crucial. These tools help identify and address bias, ensuring AI promotes equality and inclusivity in the workplace.
Transparency
Transparency in responsible AI development is paramount. A thorough comprehension of AI mechanisms is essential to foster trust and accountability. By understanding how AI arrives at decisions, both employers and employees are empowered, enhancing their reasoning abilities and ensuring outcomes free from errors or biases.
Intellectual Property
The question of original creatorship arises when AI is employed to produce artistic works. Unlike humans, AI systems are not acknowledged as legal creators. Determining ownership hinges on the creator of the AI system. However, complexities arise when individuals contribute their creativity to AI-generated content without retaining ownership rights. Therefore, meticulous attention and clear ownership policies are essential to address this issue.
AI Workplace Governance
The integration of AI in the workplace promises efficiency, productivity, and innovation. However, alongside these benefits lie challenges that require careful consideration. A recent Deloitte survey underscores this concern, revealing that 70% of HR leaders are worried about the ethical implications of AI in the workplace. This section will delve into these challenges and explore strategies to ensure responsible AI integration that fosters trust and maximizes benefits for all stakeholders.
Employee Monitoring
Employers are seen monitoring their employees using AI-powered tools which have raised privacy concerns significantly. This could lead to a distrustful work environment, data misuse, or biased decision-making. Employers need to find balance by defining the purpose of monitoring, seeking employee consent, ensuring transparency and data security, and respecting employee rights to privacy.
Decision-Making
AI algorithms give results based on the data it is trained on. For instance, if a certain algorithm is created to favor specific types of resumes in the hiring process, then it will discriminate against qualified candidates, raising ethical concerns. The complexity of algorithms will further create difficulty in ascertaining how AI has got certain results making it tough to hold anyone accountable. Businesses need to define human oversight mechanisms, focus on fairness, and establish clear accountability for AI-driven decisions.
Job displacement and reskilling
Automation driven by AI is projected to significantly impact the workforce. A 2022 McKinsey Global Institute report estimates that automation could displace up to 800 million jobs globally by 2030. This raises concerns about job security, retraining needs, and potential income inequality. Companies have a responsibility to communicate transparently about automation and its impact on the workforce. Proactive measures like reskilling initiatives can help equip employees with the necessary skills to navigate the changing job landscape. Additionally, fair practices like severance packages and assistance with job searches can ease anxieties and ensure a smoother transition for displaced workers.
AI Data Privacy and Ethical Guidelines
Global Landscape
When it comes to AI Data privacy regulations there’s no one size fits all as every country has its own priorities where some focus on guarding individual rights and some on economic security. The number of global data privacy regulations is expected to reach 100 by 2023, highlighting the increasingly complex regulatory landscape. Businesses need to understand the AI data privacy regulations of the region they are operating in and comply with multiple standards for data gathering, storage, and use.
Evolving AI Regulations
AI technology is evolving, and businesses and regulatory authorities need to establish laws and regulations that catch up with new technological advancements and potential risks. Staying abreast with the latest developments and adapting to changes will be a great step towards long-term compliance.
Ethical Guidelines
A 2023 IBM study found that 80% of consumers are concerned about how companies use their data for AI. AI ethical guidelines include laws and frameworks to guard against such negative consequences like loss of privacy or discrimination. Businesses need to ensure responsible AI development through important frameworks like the Montreal Declaration for Responsible AI Development that promote equity, justice, autonomy, and privacy.
AI Regulations: A Global, Collaborative Effort
AI regulations are emerging at various levels, creating a patchwork landscape for businesses to navigate. More than 70 countries have adopted or are developing AI-specific regulations, according to the OECD.
Well, The global AI governance market is expected to be fragmented due to varied regulations, creating compliance challenges for businesses operating across borders.
Some key trends include:
1. National regulations
The EU’s AI Act, the US AI Bill of Rights, and China’s AI ethical guidelines are examples of national efforts to regulate AI.
2. Enforcing Transparency and Explainability
The proposed European Union’s AI Act is a great initiative in the direction of transparency where companies are required to disclose how their algorithms work. This disclosure into AI working ensures accountability and prevents discrimination. The act categorizes AI applications based on risk and imposes varying compliance requirements, focusing on safety, fairness, and transparency.
3. Protecting Data Privacy
The California Consumer Privacy Act (CCPA) and General Data Protection Regulation (GDPR) are to protect individuals’ right to control their data used in AI systems.
4. Mitigating Bias
The Algorithmic Justice League is for unbiased audits and fairness frameworks to ensure equality and inclusivity.
5. Addressing Job Displacement
The World Economic Forum proposes upskilling initiatives and social safety nets to address job displacement issues after AI automation takes place.
6. US AI Bill of Rights Act
This proposed federal legislation focuses on individual rights and protections while advocating for transparency in AI algorithms, data minimization, and protection from discrimination due to biased AI outcomes.
7. California’s Automated Decision-Making Act (ADMA)
This law requires businesses using AI in high-risk situations like employment or credit decisions to explain the outcome and an opportunity for human review. While hailed as a pioneering effort in explainability and accountability, it’s criticized for being limited in scope and lacking clarity on specific implementations.
KEY TAKEAWAYS
- Establishing clear lines of responsibility is key to mitigating legal risks.
- New legal frameworks, laws, and approaches are required to address rising issues considering technological advancements and capabilities.
- Businesses need to identify potential legal risks associated with AI use with the help of AI experts, and lawyers specializing in cyber law and data privacy.
- Organizations are required to implement regulations and frameworks to ensure AI legal compliance considering the region they are operating in.
- Following AI ethical guidelines for responsible AI development and deployment.
Looking Forward
Regulating AI is a never-ending process that will go through upgrades and alterations. However, to develop effective frameworks we need:
- International Cooperation
- Multi-stakeholder Engagement
- Continuous Learning and Adaptation
Addressing these issues and opening channels for open dialogue will help us build a future where AI benefits everyone, upholding ethics and responsible AI development.
AI presents a wealth of opportunities, but responsible development is the need of the hour. Businesses need to address concerns related to AI legal compliance, data privacy, and ethical principles, so they can create a trustworthy workplace. This journey starts with education, where AI CERTs™ can help professionals and entrepreneurs upskill in the AI landscape. Explore different certifications that suit your experience, area of interest, and background and join hands for responsible AI development.