
Prince Thakur
6 months ago
Transforming data/model governance using AI and machine learning
Artificial Intelligence (AI) is omnipresent, affecting every part of our daily lives, whether personal or professional. From digital voice assistants to chatbots and monitoring of credit card fraud, AI is present everywhere. It also leads to the accumulation of data through different sources, making data and AI governance more relevant. However, the effectiveness of AI and machine learning (ML) applications depends on the quality of the data fed to their algorithms.
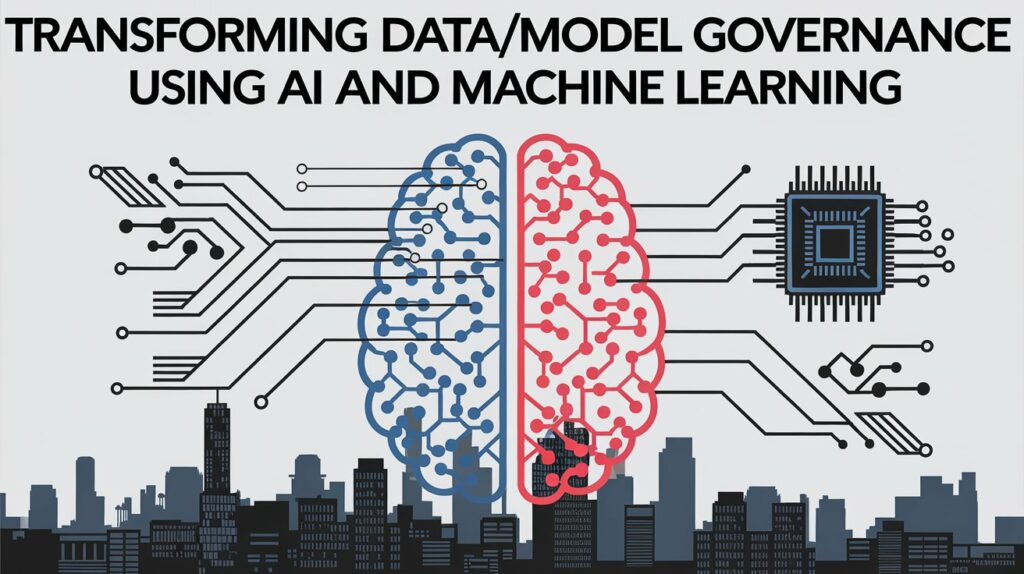
Several instances have resulted in incorrect outcomes due to flawed, biased, and inaccurate data. Surveys suggest that about 65% of business executives are worried about data bias in their companies, and 13% are working to address it. There is also an apprehension that data bias will become a bigger concern with the higher adoption of AI technologies.
Before we dive in, let's first understand data bias, which is a term used to refer to data that is incomplete or inaccurate. This leads to systematic errors in AI and ML applications, because of which they fail to present an accurate picture of the required information due to the inaccurate data they rely on. This data bias can come from various data sources, including the selection of data, the methods of data gathering, and the algorithms used to analyse the data. When the data used in AI and ML training processes is unrepresentative, inaccurate, or flawed, it can distort results, leading to decisions that support existing inequalities or produce incorrect or undesirable outcomes. In AI systems, this bias can be seen in many ways, impacting everything—not only recommendations and results but also predictions and categorisation.
In the health care industry, it has been reported that medical data concerning women and minority populations is inadequately represented. One example is the lower diagnostic accuracy of AI systems for black patients compared to their white counterparts. Similarly, in the fields of recruitment and talent acquisition, AI systems utilising natural language processing (NLP) have shown biased outcomes. A notable example is Amazon's AI recruitment tool, which was abandoned after it demonstrated a preference for candidates whose resumes contained certain action verbs.
A recent study uncovered bias in Midjourney, a generative AI image-creation tool. When tasked with producing images of professionals across various age groups, the application showed diversity in age but not in gender for older individuals. Specifically, all depictions of senior professionals were male, perpetuating stereotypes about gender roles in the workforce. Another study revealed gender-based disparities in online job advertisements distributed by search engines. A study conducted by Carnegie Mellon University discovered that an internet advertising platform was more likely to present high-paying job opportunities to male users compared to female users.