
Prince Thakur
5 months ago
Liquid AI debuts new LFM-based models that seem to outperform most traditional large language models
Artificial intelligence startup and MIT spinoff Liquid AI Inc. today launched its first set of generative AI models, and they’re notably different from competing models because they’re built on a fundamentally new architecture. The new models are being called “Liquid Foundation Models,” or LFMs, and they’re said to deliver impressive performance that’s on a par with, or even superior to, some of the best large language models available today.
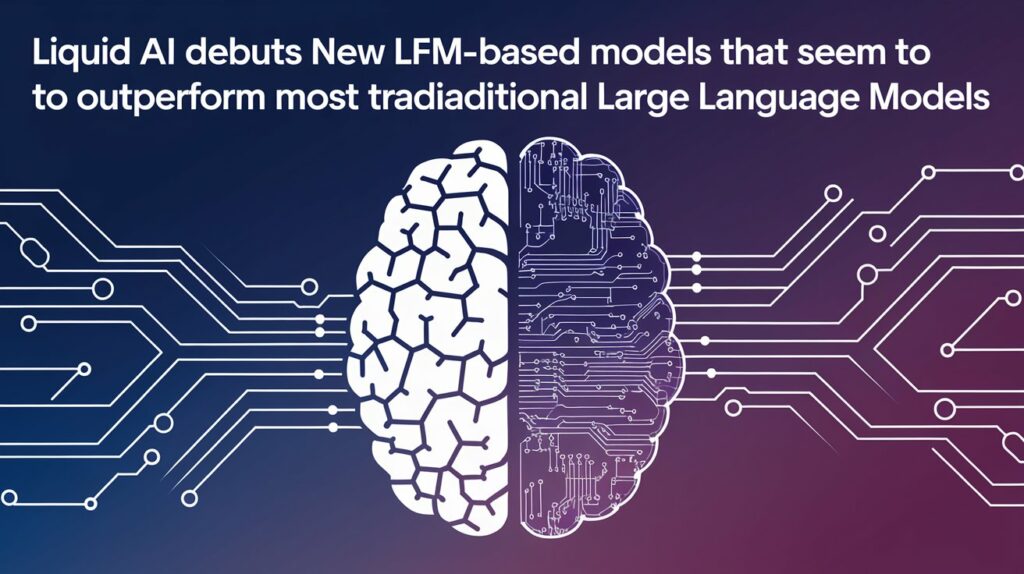
The Boston-based startup was founded by a team of researchers from the Massachusetts Institute of Technology, including Ramin Hasani, Mathias Lechner, Alexander Amini and Daniela Rus. They’re said to be pioneers in the concept of “liquid neural networks,” which is a class of AI models that’s quite different from the Generative Pre-trained Transformer-based models we know and love today, such as OpenAI’s GPT series and Google LLC’s Gemini models.
The company’s mission is to create highly capable and efficient general-purpose models that can be used by organizations of all sizes. To do that, it’s building LFM-based AI systems that can work at every scale, from the network edge to enterprise-grade deployments.
What are LFMs?
According to Liquid, its LFMs represent a new generation of AI systems that are designed with both performance and efficiency in mind. They use minimal system memory while delivering exceptional computing power, the company explains.
They’re grounded in dynamical systems, numerical linear algebra and signal processing. That makes them ideal for handling various types of sequential data, including text, audio, images, video and signals.
Liquid AI first made headlines in December when it raised $37.6 million in seed funding. At the time, it explained that its LFMs are based on a newer, Liquid Neural Network architecture that was originally developed at MIT’s Computer Science and Artificial Intelligence Laboratory. LNNs are based on the concept of artificial neurons, or nodes for transforming data.
Whereas traditional deep learning models need thousands of neurons to perform computing tasks, LNNs can achieve the same performance with significantly fewer. It does this by combining those neurons with innovative mathematical formulations, enabling it to do much more with less.
The startup says its LFMs retain this adaptable and efficient capability, which enables them to perform real-time adjustments during inference without the enormous computational overheads associated with traditional LLMs. As a result, they can handle up to 1 million tokens efficiently without any noticeable impact on memory usage.