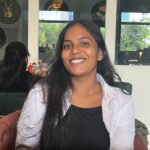
Aishwarya Nair
5 months ago
How AI is Improving Simulations with Smarter Sampling Techniques
Artificial intelligence (AI) is revolutionizing simulations by introducing smarter sampling techniques that enhance efficiency and accuracy. In industries like aerospace, healthcare, and finance, simulations play a vital role in modeling complex systems, but traditional methods often demand extensive computational resources. AI-driven techniques like machine learning, active learning, and Bayesian optimization are enabling simulations to run faster by focusing on critical scenarios, reducing the need for unnecessary samples. This advancement is transforming industries by delivering more accurate results while cutting down on time and resources required for simulations.
Artificial intelligence (AI) is transforming industries across the globe, and one area where it’s making a significant impact is in improving simulations through smarter sampling techniques. From engineering and scientific research to finance and healthcare, simulations play a crucial role in modeling real-world processes. However, traditional simulation methods often require enormous computational resources and time. AI is changing this by enabling more efficient simulations through smarter, faster, and more precise sampling techniques, revolutionizing various fields.
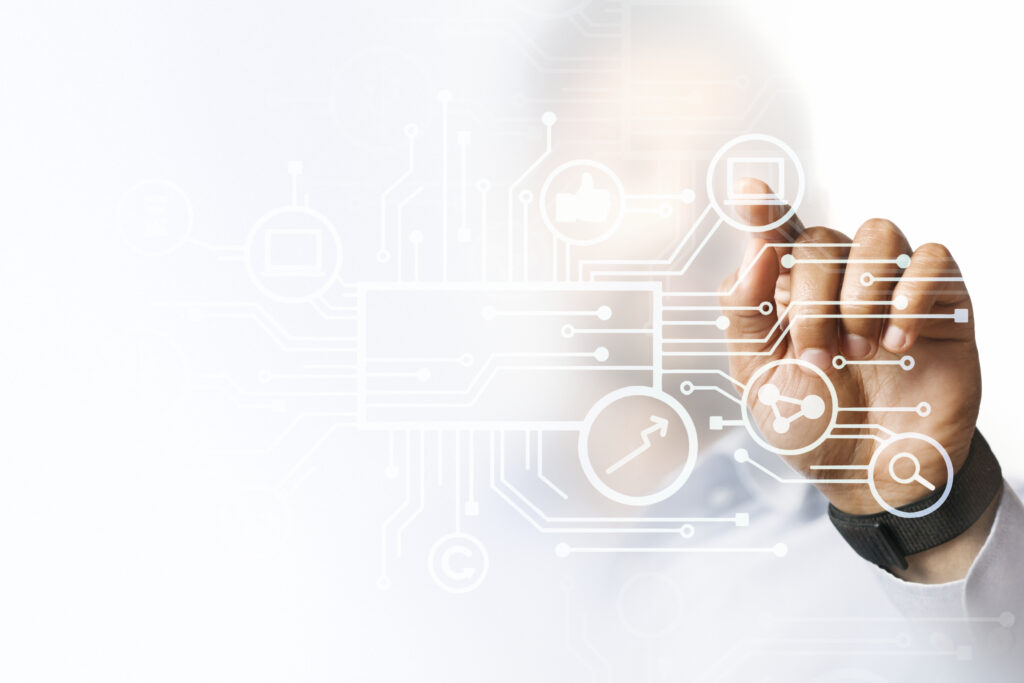
The Importance of Simulations
Simulations are used to model complex systems, predict outcomes, and test different scenarios without the need for physical trials. In industries like aerospace, automotive, and pharmaceuticals, simulations are essential for testing designs, optimizing processes, and predicting behaviors under varying conditions. However, traditional simulation methods can be time-consuming and resource-intensive because they often rely on random or brute-force sampling techniques that may not efficiently capture the full range of possible outcomes.
This is where AI is stepping in to enhance the process.
Smarter Sampling with AI
AI-powered algorithms have introduced smarter sampling techniques that dramatically improve the efficiency and accuracy of simulations. These techniques involve the use of machine learning models to identify patterns and optimize the selection of sample points, enabling simulations to run faster and with fewer resources.
- Machine Learning-Driven Sampling
- One of the primary AI techniques for transforming simulations is machine learning (ML). By learning from previous simulation results, ML models can predict which areas of the simulation space are most important to sample. This allows simulations to focus on the most critical scenarios rather than wasting resources on areas of lesser importance. The result is faster simulations without compromising accuracy.
- Active Learning
Active learning, a subset of machine learning, further enhances sampling by iteratively selecting the most informative data points for training models. In simulations, this means the algorithm can intelligently choose the next best sample point based on prior knowledge. This approach reduces the number of samples needed while increasing the quality of the simulation's predictions. For example, in climate modeling or drug discovery, active learning can help narrow down the search space for potential outcomes, making the simulations far more efficient.
- Bayesian Optimization
Bayesian optimization is another AI-driven technique improving simulations. It uses a probabilistic model to guide sampling, focusing on the most promising regions of the simulation space. This approach balances exploration and exploitation, helping simulations zero in on optimal solutions faster. In industries like engineering or finance, where simulations are used to model complex systems with many variables, Bayesian optimization offers a powerful tool for achieving faster, more accurate results.
Benefits Across Industries
The integration of AI into simulation processes is already delivering substantial benefits across various industries:
- Aerospace and Automotive: In these sectors, AI-enhanced simulations are optimizing design and safety testing, reducing the need for physical prototypes and enabling faster innovation cycles.
- Healthcare: AI-powered simulations in drug discovery and personalized medicine are accelerating the development of treatments by optimizing which compounds to test in clinical trials.
- Finance: Simulations in financial markets are used to model economic scenarios, forecast risks, and optimize trading strategies. AI improves the accuracy of these simulations by reducing unnecessary computational overhead.
Addressing Challenges
While AI is revolutionizing simulations, it’s important to acknowledge the challenges that come with it. Data quality is a critical factor—poor-quality data can lead to inaccurate AI models and faulty simulations. Additionally, there are concerns about transparency, as some AI models, particularly deep learning models, can be seen as "black boxes," making it difficult to understand how they arrive at specific conclusions.
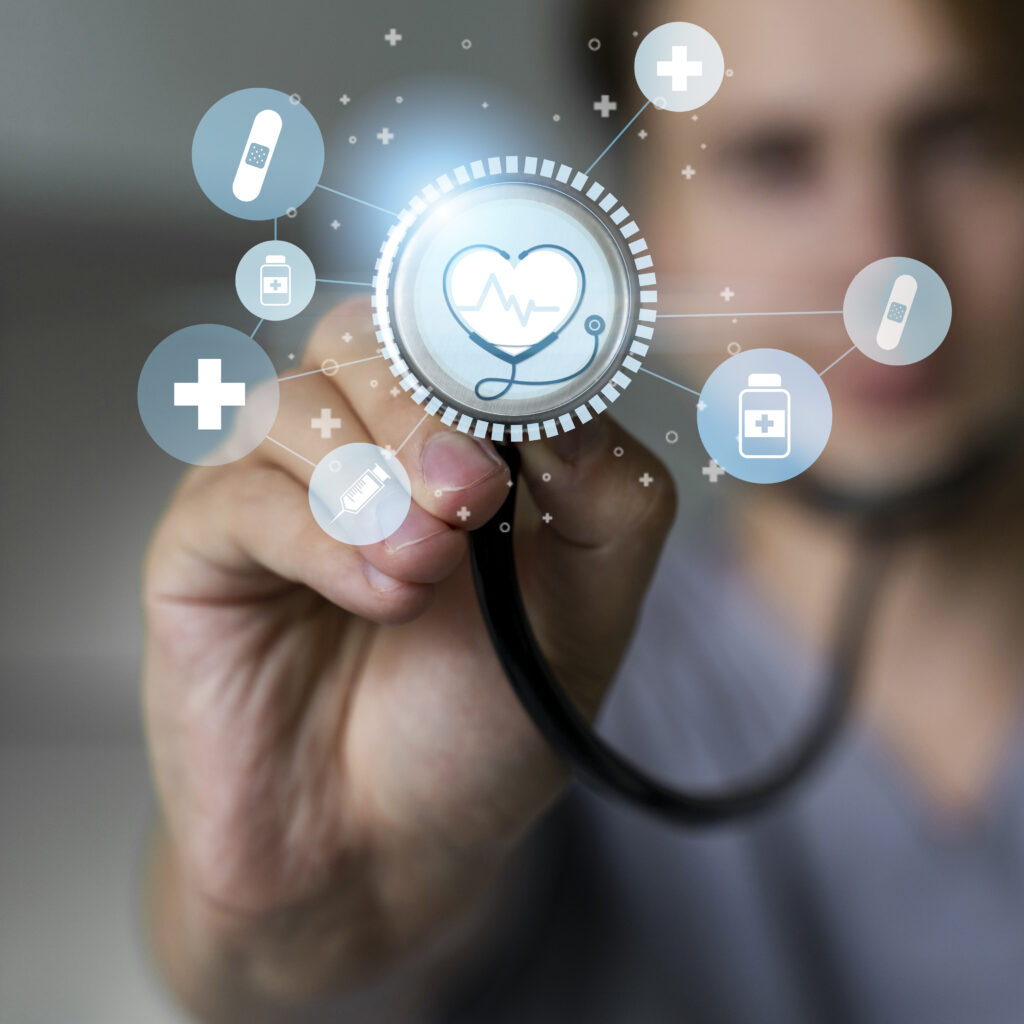
Conclusion: The Future of Simulations with AI
AI-driven smarter sampling techniques are transforming simulations across industries, providing faster, more efficient, and more accurate results. As AI continues to advance, the potential for further improvements in simulations is vast, promising to unlock new possibilities in fields ranging from engineering to medicine. For businesses and researchers, adopting AI-enhanced simulations will be key to staying competitive and driving innovation in an increasingly data-driven world.
By leveraging smarter AI-powered sampling techniques, simulations can push the boundaries of what’s possible, reducing time and resource requirements while ensuring precise and actionable insights. Read more.