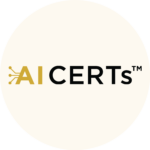
AI CERTS
15 hours ago
AI System Predicts Protein Fragments for Target Binding and Inhibition
Researchers at the Massachusetts Institute of Technology (MIT) have developed an innovative AI system capable of accurately predicting protein fragments that can bind to or inhibit specific targets. This advancement holds significant promise for accelerating drug discovery and enhancing the precision of therapeutic interventions.
In a recent study, MIT scientists introduced an AI-based tool named FragFold, designed to predict fragment inhibitors—a novel application of the AlphaFold system. FragFold analyzes protein structures to identify potential binding sites and suggests peptide sequences that can effectively interact with disease-related targets. Experimental validation demonstrated that more than half of FragFold's predictions were accurate, even in cases lacking prior structural data on the interactions.
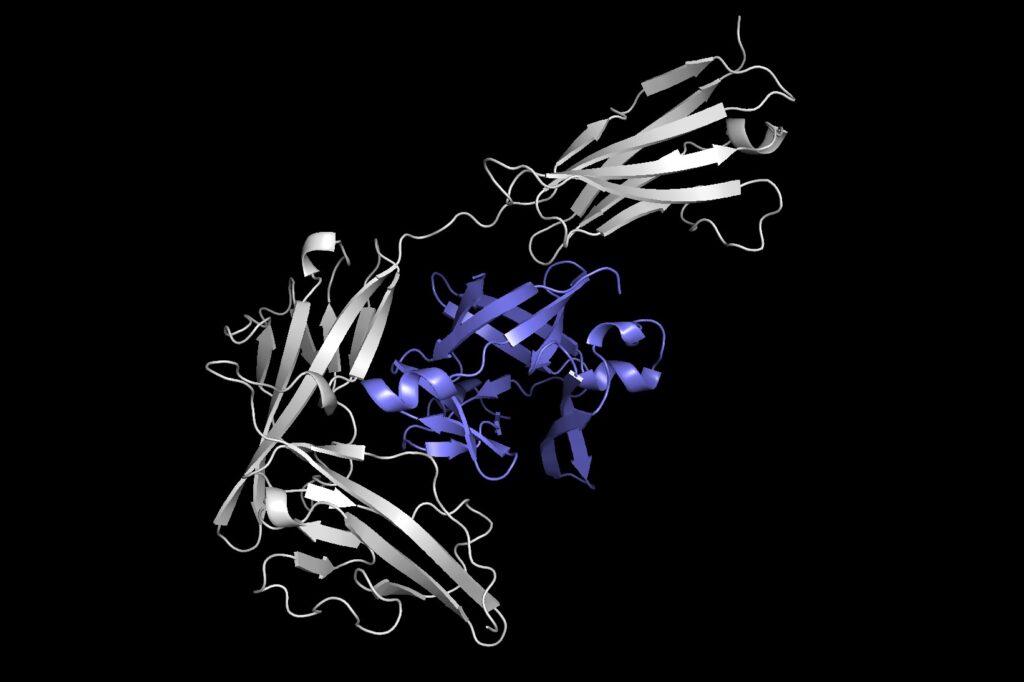
This AI-driven approach streamlines the identification of protein-ligand interactions, a critical aspect of drug development. Traditional methods of determining protein-ligand binding affinity are often time-consuming and costly. The integration of deep learning techniques, as seen in FragFold, offers a more efficient pathway by rapidly analyzing complex protein structures and predicting their interaction potentials.
The implications of this technology are profound, potentially leading to the development of targeted therapies for a range of diseases, including cancer, neurodegenerative disorders, and infectious diseases. By accurately predicting how small protein fragments interact with specific targets, researchers can design more effective inhibitors, thereby enhancing treatment efficacy and reducing adverse effects.
While the promise of AI in protein interaction prediction is evident, experts emphasize the need for further research to refine these models and ensure their applicability across diverse biological systems. Collaborative efforts between computational scientists and experimental biologists will be essential to fully harness the potential of AI in therapeutic development.
Sources-
https://link.springer.com/article/10.1007/s12551-022-01032-7