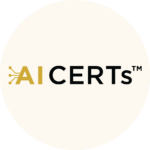
AI CERTS
4 months ago
AI Academic Research Certification: Unlocking the Power of Artificial Intelligence in Scholarship
Artificial Intelligence (AI) has become an indispensable tool in academic research, enabling breakthroughs in data processing, pattern recognition, and predictive modeling. Scholars across disciplines are leveraging AI to address complex questions, enhance methodologies, and accelerate discoveries. An AI academic research certification equips researchers with the knowledge and skills to integrate AI technologies into their work effectively.
This article explores in detail the importance of AI in academic research, the key competencies developed through certification, real-world applications, challenges, and strategies for successful integration of AI tools.
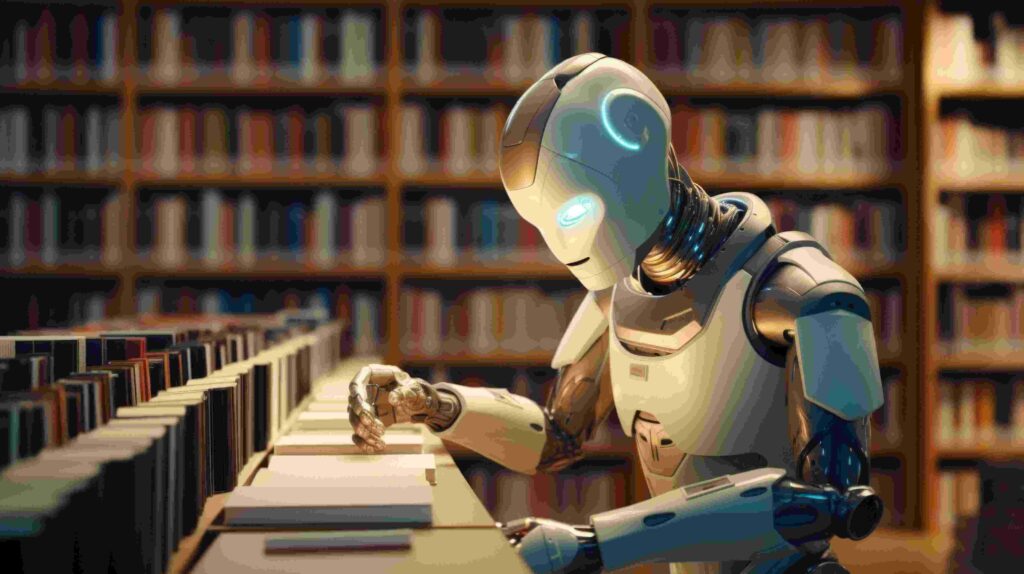
Importance of AI in Academic Research
AI’s role in academic research extends beyond automation; it facilitates deep insights and fosters innovation.
Enhancing Efficiency in Research
AI reduces the time researchers spend on repetitive tasks, such as data entry and basic analysis, enabling them to focus on complex problem-solving and interpretation.
Improving Data Analysis
AI algorithms analyze vast datasets quickly and accurately, identifying patterns and correlations that traditional methods might overlook. For example, in genomic studies, AI helps identify gene expressions linked to diseases.
Encouraging Interdisciplinary Collaboration
AI acts as a bridge between fields such as computer science, biology, and linguistics, fostering interdisciplinary research.
Supporting Predictive and Prescriptive Analysis
By utilizing machine learning models, researchers can predict outcomes and prescribe solutions, advancing fields like environmental science and epidemiology.
External Resource: Explore AI’s impact on research at Nature AI and Research Insights.
Skills Gained from AI Academic Research Certification
An AI academic research certification provides comprehensive training in technical and methodological aspects:
1. AI Fundamentals
Learn the basics of AI, machine learning, and deep learning, including how these technologies apply to research contexts.
2. Data Preparation and Analysis
Develop expertise in cleaning, organizing, and analyzing datasets, ensuring high-quality inputs for AI models.
3. Model Development
Master the creation, training, and optimization of machine learning models tailored to specific research objectives.
4. Visualization Techniques
Learn to present findings effectively through advanced visualization tools and software.
5. Ethics and Fairness in AI
Understand the ethical implications of AI in research, including bias mitigation, data privacy, and transparency.
External Resource: For a beginner’s guide to AI ethics, visit 9 ethical AI principles for organizations to follow at World economic forum
Real-World Applications of AI in Academic Research
1. Natural Sciences
AI accelerates discoveries in physics, chemistry, and biology. For instance, AI-driven simulations predict material properties, aiding in the design of new compounds.
2. Social Sciences
Researchers use AI to analyze societal trends through social media, survey responses, and historical data, uncovering insights into human behavior.
3. Healthcare and Medicine
AI models assist in genomic analysis, disease diagnosis, and drug discovery, expediting critical research and improving patient outcomes.
4. Climate Science
AI predicts environmental changes and identifies sustainable solutions, helping address climate challenges effectively.
External Resource: Discover AI’s applications in scientific discovery at IBM
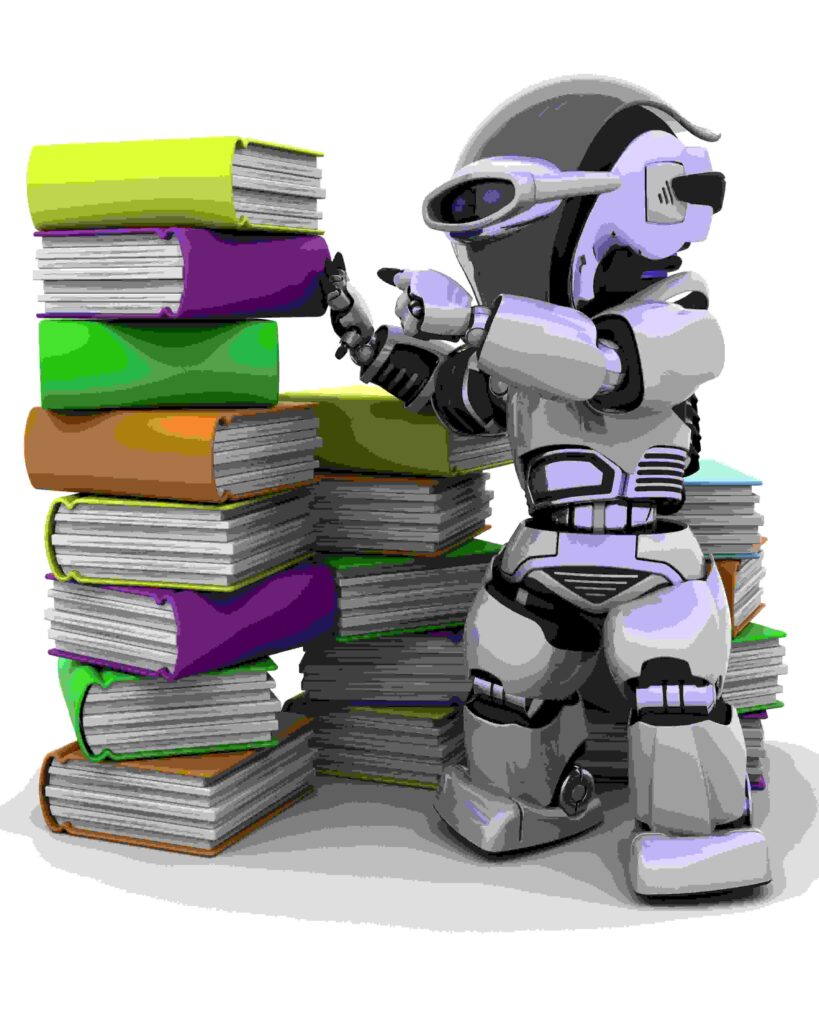
Challenges in Integrating AI into Academic Research
1. Data Accessibility
Many researchers face difficulties accessing high-quality datasets, especially in resource-constrained environments.
2. Complexity of AI Tools
AI models require significant technical knowledge, posing a barrier for researchers without prior expertise in data science.
3. Bias in AI Models
Algorithmic biases can skew research outcomes, necessitating robust evaluation and validation techniques.
4. Ethical Considerations
Ensuring transparency, accountability, and compliance with data protection laws like GDPR is crucial in AI-driven research.
Strategies for Excelling in AI-Powered Research
1. Continuous Learning
Stay updated on the latest AI advancements through webinars, conferences, and journals.
2. Collaboration
Engage with interdisciplinary teams to enrich perspectives and access diverse skill sets.
3. Invest in Infrastructure
Leverage cloud computing and open-source tools to reduce resource constraints.
4. Ethics-First Approach
Adopt ethical AI practices to build trust and ensure equitable research outcomes.
External Resource: For open-source AI tools, visit Pesto Tech
Future Trends in AI Academic Research
The future of AI in academic research is bright, with several emerging trends:
- AI-Driven Literature Review: Tools like Semantic Scholar use NLP to streamline the review of academic papers.
- Explainable AI (XAI): Focus on creating models that provide transparent and interpretable results.
- Cross-Language Research: NLP technologies break language barriers, enabling global collaboration.
- Automation of Experimental Design: AI tools will increasingly support hypothesis generation and experiment planning.
Conclusion
An AI academic research certification equips scholars with the skills to navigate the evolving landscape of AI-powered research. By mastering AI tools and methodologies, researchers can accelerate discoveries, foster interdisciplinary collaboration, and contribute to knowledge creation in unprecedented ways.
We can also take AI+ Researcher™ certification course in reputed company like AICERTs. Use the coupon code NEWCOURSE25 to get 25% OFF on AI CERTS' certifications. Don't miss out on this limited-time offer! Visit this link to explore AI+ Researcher™ certification and enroll today.